布隆过滤器的方式解决缓存穿透问题
原理
布隆过滤器的巨大用处就是,能够迅速判断一个元素是否在一个集合中。因此他有如下三个使用场景:
- 网页爬虫对URL的去重,避免爬取相同的URL地址
- 反垃圾邮件,从数十亿个垃圾邮件列表中判断某邮箱是否垃圾邮箱(同理,垃圾短信)
- 缓存穿透,将所有可能存在的数据缓存放到布隆过滤器中,当黑客访问不存在的缓存时迅速返回避免缓存及DB挂掉
OK,接下来我们来谈谈布隆过滤器的原理
其内部维护一个全为0的bit数组,需要说明的是,布隆过滤器有一个误判率的概念,误判率越低,则数组越长,所占空间越大。误判率越高则数组越小,所占的空间越小。
假设,根据误判率,我们生成一个10位的bit数组,以及2个hash函数(f_1,f_2)
,如下图所示(生成的数组的位数和hash函数的数量,我们不用去关心是如何生成的,有数学论文进行过专业的证明)。
假设输入集合为((N_1,N_2))
,经过计算(f_1(N_1))
得到的数值得为2,(f_2(N_1))
得到的数值为5,则将数组下标为2和下表为5的位置置为1,如下图所示
同理,经过计算(f_1(N_2))
得到的数值得为3,(f_2(N_2))
得到的数值为6,则将数组下标为3和下表为6的位置置为1,如下图所示
这个时候,我们有第三个数(N_3)
,我们判断(N_3)
在不在集合((N_1,N_2))
中,就进行(f_1(N_3),f_2(N_3))
的计算
- 若值恰巧都位于上图的红色位置中,我们则认为,
(N_3)
在集合((N_1,N_2))
中 - 若值有一个不位于上图的红色位置中,我们则认为,
(N_3)
不在集合((N_1,N_2))
中
以上就是布隆过滤器的计算原理,下面我们进行性能测试,
性能测试
(1) 新建一个maven工程,引入guava包
<dependencies>
<dependency>
<groupId>com.google.guava</groupId>
<artifactId>guava</artifactId>
</dependency>
</dependencies>
(2) 测试一个元素是否属于一个百万元素集合所需耗时
import java.util.ArrayList;import java.util.List;
import com.google.common.hash.BloomFilter;
import com.google.common.hash.Funnels;
public class Test {
private static int size = 1000000;
private static BloomFilter<Integer> bloomFilter = BloomFilter.create(Funnels.integerFunnel(), size);
public static void main(String[] args) {
for (int i = 0; i < size; i++) {
bloomFilter.put(i);
}
List<Integer> list = new ArrayList<Integer>(1000);
//故意取10000个不在过滤器里的值,看看有多少个会被认为在过滤器里
for (int i = size + 10000; i < size + 20000; i++) {
if (bloomFilter.mightContain(i)) {
list.add(i);
}
}
System.out.println("误判的数量:" + list.size());
}
}
输出结果如下
误判对数量:330
如果上述代码所示,我们故意取10000个不在过滤器里的值,却还有330个被认为在过滤器里,这说明了误判率为0.03.即,在不做任何设置的情况下,默认的误判率为0.03。
下面上源码来证明:
/**
* Creates a {@link BloomFilter BloomFilter<T>} with the expected number of
* insertions and a default expected false positive probability of 3%.
*
* <p>Note that overflowing a {@code BloomFilter} with significantly more elements
* than specified, will result in its saturation, and a sharp deterioration of its
* false positive probability.
*
* <p>The constructed {@code BloomFilter<T>} will be serializable if the provided
* {@code Funnel<T>} is.
*
* <p>It is recommended that the funnel be implemented as a Java enum. This has the
* benefit of ensuring proper serialization and deserialization, which is important
* since {@link #equals} also relies on object identity of funnels.
*
* @param funnel the funnel of T's that the constructed {@code BloomFilter<T>} will use
* @param expectedInsertions the number of expected insertions to the constructed
* {@code BloomFilter<T>}; must be positive
* @return a {@code BloomFilter}
* @since 19.0
*/
@CheckReturnValue
public static <T> BloomFilter<T> create(Funnel<? super T> funnel, long expectedInsertions) {
return create(funnel, expectedInsertions, 0.03); // FYI, for 3%, we always get 5 hash functions
}
接下来我们通过调试查看numBits
和numHashFunctions
:
@VisibleForTesting
static <T> BloomFilter<T> create(
Funnel<? super T> funnel, long expectedInsertions, double fpp, Strategy strategy) {
checkNotNull(funnel);
checkArgument(
expectedInsertions >= 0, "Expected insertions (%s) must be >= 0", expectedInsertions);
checkArgument(fpp > 0.0, "False positive probability (%s) must be > 0.0", fpp);
checkArgument(fpp < 1.0, "False positive probability (%s) must be < 1.0", fpp);
checkNotNull(strategy);
if (expectedInsertions == 0) {
expectedInsertions = 1;
}
/*
* TODO(user): Put a warning in the javadoc about tiny fpp values,
* since the resulting size is proportional to -log(p), but there is not
* much of a point after all, e.g. optimalM(1000, 0.0000000000000001) = 76680
* which is less than 10kb. Who cares!
*/
long numBits = optimalNumOfBits(expectedInsertions, fpp);
int numHashFunctions = optimalNumOfHashFunctions(expectedInsertions, numBits);
try {
return new BloomFilter<T>(new BitArray(numBits), numHashFunctions, funnel, strategy);
} catch (IllegalArgumentException e) {
throw new IllegalArgumentException("Could not create BloomFilter of " + numBits + " bits", e);
}
}
来看一下,误判率为0.03时,底层维护的bit数组的长度和hash函数数量:
numBits = 7298000
numHashFunctions = 5
将bloomfilter的构造方法改为
private static BloomFilter<Integer> bloomFilter = BloomFilter.create(Funnels.integerFunnel(), size, 0.01);
即,此时误判率为0.01。在这种情况下,底层维护的bit数组的长度和hash函数数量:
numBits = 9585000
numHashFunctions = 7
由此可见,误判率越低,则底层维护的数组越长,占用空间越大。因此,误判率实际取值,根据服务器所能够承受的负载来决定,不是拍脑袋瞎想的。
实际使用
Redis伪代码如下所示
String get(String key) {
String value = redis.get(key);
if (value == null) {
if (!bloomfilter.mightContain(key)) {
return null;
} else {
bloomFilter.put(key);
value = db.get(key);
redis.set(key, value);
}
}
return value;
}
(1)优点
- 思路简单
- 保证一致性
- 性能强
(2)缺点
- 代码复杂度增大
- 需要另外维护一个集合来存放缓存的Key
- 布隆过滤器不支持删值操作
版权声明:
作者:Joe.Ye
链接:https://www.appblog.cn/index.php/2023/04/02/solving-cache-penetration-issues-through-blossom-filter/
来源:APP全栈技术分享
文章版权归作者所有,未经允许请勿转载。
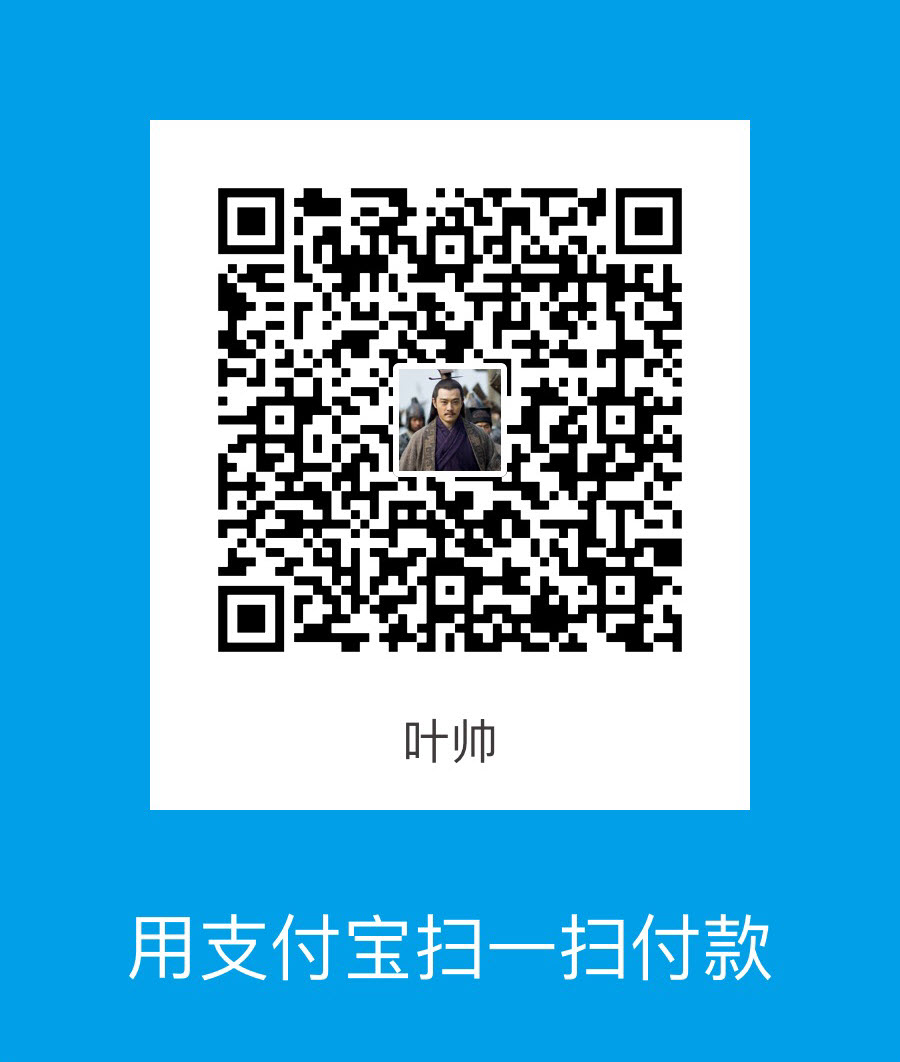
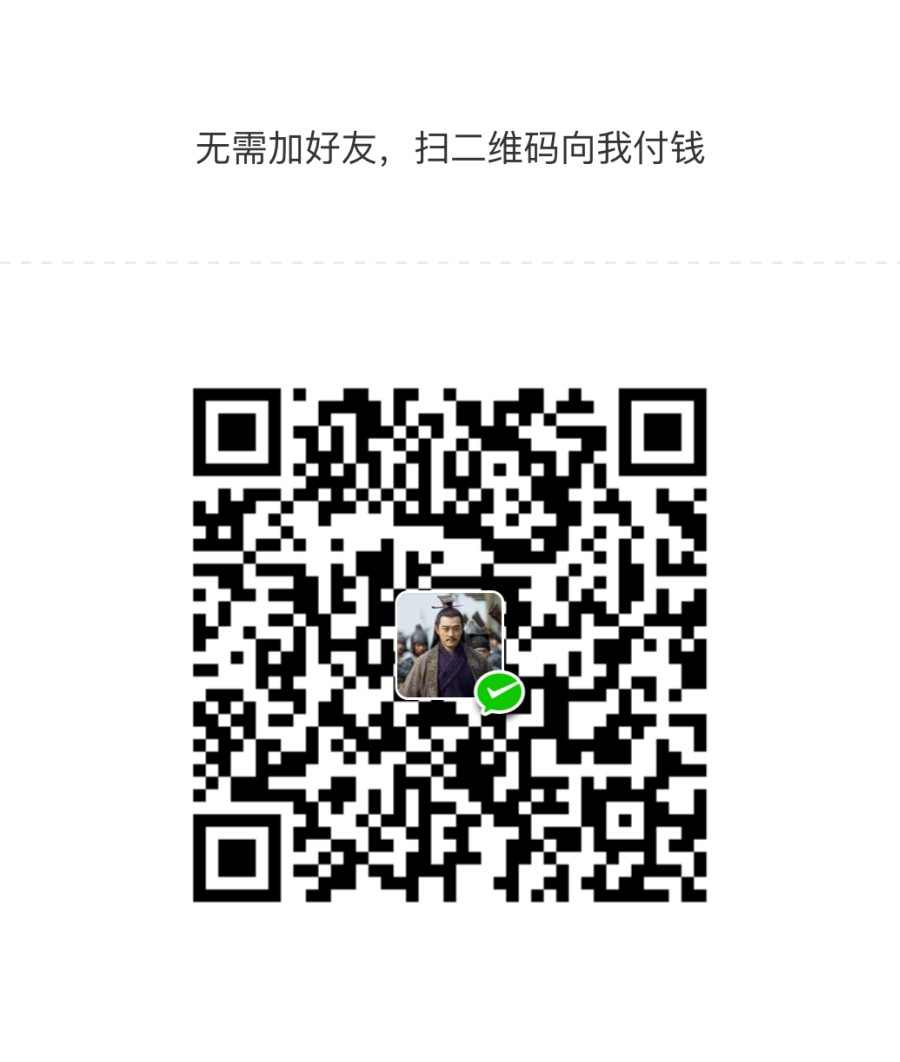
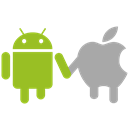
共有 0 条评论